CESNET scientists publish unique dataset in Nature Scientific Data
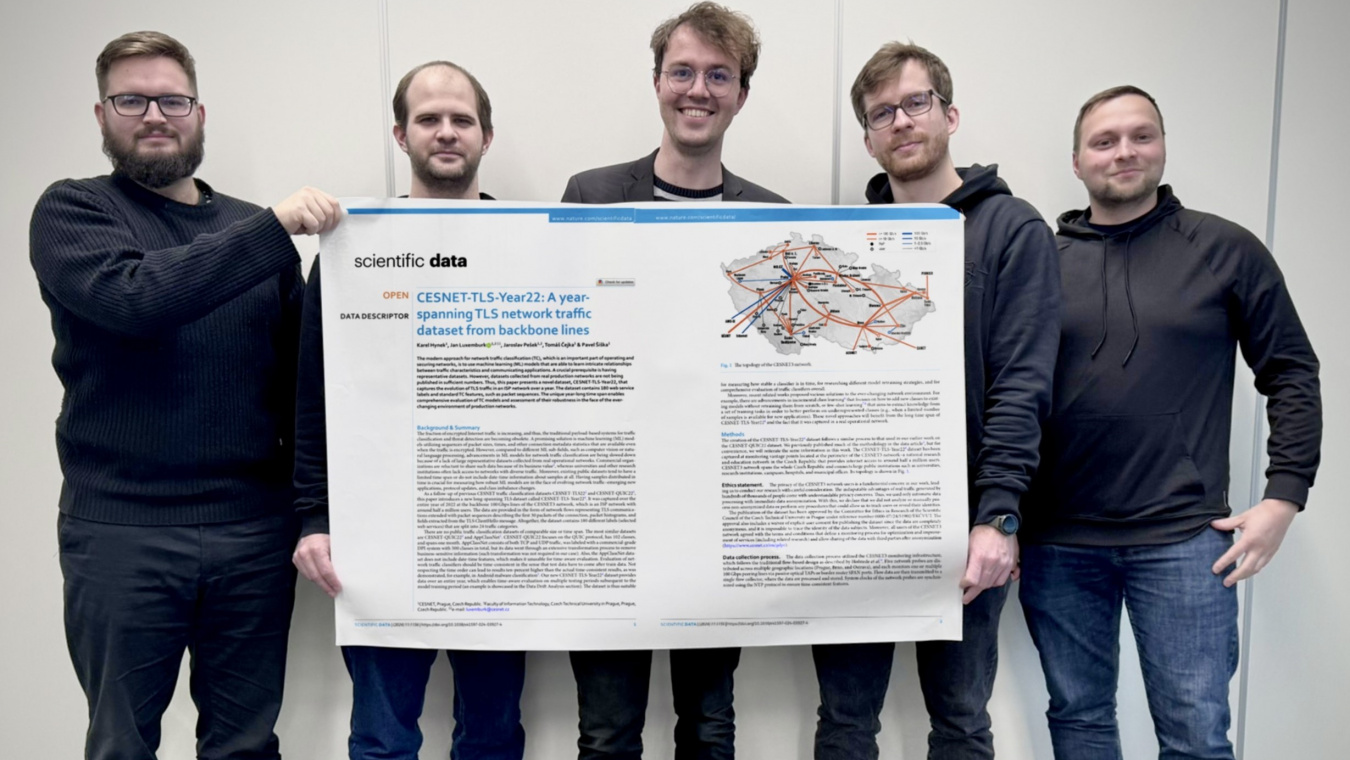
Researchers from CESNET's Administration and Security Tools Division have created and published a dataset that is a valuable tool for understanding dynamic changes in network traffic. This unique dataset represents a major step in addressing cyber threats and its uniqueness has earned it publication in the prestigious journal Nature Scientific Data.
The importance of machine learning models for detecting security threats on computer networks has long been known to both the scientific and professional communities. CESNET researchers are investigating the use of machine learning methods over network traffic in the project "Analysis of encrypted traffic using network flows", which was selected among the supported projects in the framework of the IMPAKT 1 call of the Ministry of Interior of the Czech Republic. Although several highly innovative and accurate machine learning detectors have already been developed during the project, their mass deployment is still hampered by several difficult-to-solve problems. One of the most frequently mentioned is the problem of so-called data shift-phenomena, where the machine learning model was developed on data that is outdated and no longer reflects the current state.
Data sets in everyday life and how they work
You may have tried to log in to your phone or computer using facial recognition (such as Apple Face ID or Windows Hello), but the device simply didn't recognise you. This happened because the system was trained on your historical appearance, which may have changed-for example, there was a slight swelling of your face due to a sleepless night, or you changed your hairstyle, which now hits your face differently. In this case, a data drift has occurred; the training data (your likeness) was out of date and the verification did not work correctly.
However, biometric facial verification effectively counteracts the data shift problem through regular re-training. Each time the device successfully verifies your face, it updates your likeness to recognize you again the next time. This system usually works because our appearance changes relatively slowly. However, if there is a sudden change (for example, if men shave), verification often fails and a backup method-password reset-needs to be activated.
The importance of datasets for the security of network traffic
A similar problem arises in cybersecurity. However, unlike most common situations, the data shift in cybersecurity is usually sudden and unpredictable. Cybercriminals may find new methods of attack, or the deployment of new services on the network may dramatically affect the nature of traffic. Even minor updates to certificates can fundamentally change the nature of network data, disrupting the functionality of machine learning.
In cybersecurity, we typically do not have backup detection methods that work 100%, so it is critical to investigate this phenomenon. Given the virtual absence of available datasets suitable for this research, researchers have had limited options until now-fortunately, a new dataset has just been created that enables this research.
A year of network traffic in a groundbreaking dataset
A team of scientists from CESNET and the Faculty of Information Technology of the Czech Technical University in Prague, consisting of Karel Hynek, Jan Luxemburk, Jaroslav Pešek, Tomáš Čejka and Pavel Šiška, has created and published a unique dataset in the prestigious journal Nature Scientific Data, which includes an entire year of anonymized network traffic from the backbone links of the national academic network. Until now, the scientific community has had datasets capturing a few days or a week, due to the difficulty of long-term collection and the volume of the overall data. The creation of a dataset containing a full year of traffic is unprecedented and therefore a crucial step in addressing challenges such as data drift and its negative impact on network traffic security.
The newly created dataset not only allows to investigate the gradually decreasing accuracy of existing algorithms, but also supports the development of new methods that will be able to adaptively respond to constantly changing conditions in network traffic. It provides researchers and network security practitioners with valuable tools for analyzing machine learning behavior in a dynamic and rapidly changing cyber threat environment. Given the rapid evolution of technologies and attack methods, it is critical that the scientific and professional community continue to research and implement effective solutions to provide protection from cyber threats and improve the overall security of the digital environment.
In this context, CESNET profiles itself as a leader in the field of network security - not only conducting cutting-edge research, but also actively creating the conditions for its implementation and supporting further development in this area. The dataset published in the prestigious journal Nature Scientific Data is one of the examples of high quality results that enables the expert community to respond effectively to current and future challenges in the field of cyber security.
You can read the full article in English here.